AI for Nonlinear Control: A Revolutionary Approach
April 18, 2025 2025-04-11 10:37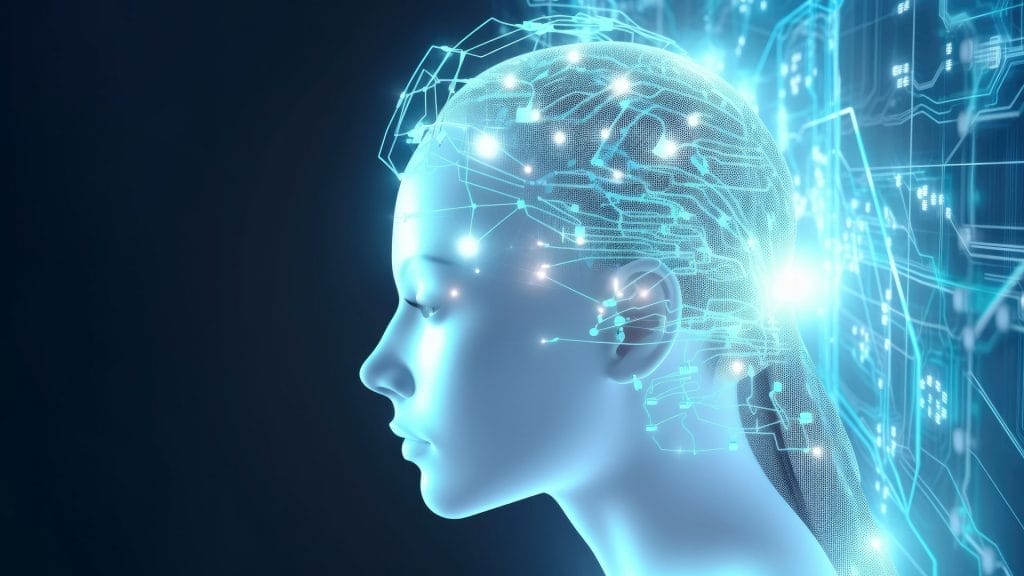
AI for Nonlinear Control: A Revolutionary Approach
In the ever-evolving world of control systems, the integration of artificial intelligence (AI) with nonlinear control techniques is rapidly transforming industries and systems engineering. Nonlinear control, which deals with the control of systems where the relationship between the inputs and outputs is not proportional, has traditionally been a challenging field. However, with the recent advancements in AI, particularly in machine learning and neural networks, the potential for nonlinear control systems has expanded dramatically.
This article explores how AI is revolutionizing nonlinear control systems, offering more efficient, adaptable, and intelligent ways to handle complex systems in various applications.
What is Nonlinear Control?
Nonlinear control refers to the control of systems where the output is not directly proportional to the input. Unlike linear systems, where the principle of superposition holds, nonlinear systems behave in a much more complex manner. This complexity can lead to challenges in stability, response time, and predictability.
For instance, systems like robots, aircraft, or climate control systems are often nonlinear in nature due to various dynamic interactions and changing conditions. Traditional control methods, which rely on linear models, may not effectively handle these types of systems. Hence, nonlinear control techniques such as feedback linearization, Lyapunov methods, and Sliding Mode Control have been developed to address these challenges.
The Role of AI in Nonlinear Control
AI, especially machine learning (ML), has introduced innovative ways to optimize nonlinear control systems. By leveraging AI’s capability to process vast amounts of data, learn patterns, and predict system behavior, engineers are now able to design smarter control systems. The integration of AI with nonlinear control offers several advantages:
1. Improved System Modeling
AI can enhance the modeling of nonlinear systems by training algorithms on real-world data. Machine learning algorithms can learn the underlying dynamics of a nonlinear system without needing an explicit mathematical model. This is particularly valuable when it is difficult or impossible to derive accurate models due to system complexity or unknown variables.
For example, neural networks can approximate complex functions that describe the system’s behavior, allowing for better prediction and control of systems like autonomous vehicles or power grids.
2. Adaptive Control
One of the key advantages of AI in nonlinear control is its ability to adapt to changing conditions. Traditional control systems rely on pre-defined rules and models that may not perform well in dynamic environments. AI-based systems, on the other hand, can continuously learn and adapt in real-time.
This ability to adapt is particularly useful in environments where external conditions constantly change, such as in robotics or aerospace systems. For example, reinforcement learning, a branch of machine learning, allows a control system to adjust its actions based on feedback from the environment, thereby improving performance over time.
3. Optimization of Control Inputs
AI techniques such as genetic algorithms and deep learning can be applied to optimize the control inputs for nonlinear systems. These algorithms can search for the best possible set of control parameters that minimize errors, energy consumption, or other performance metrics. AI can help find solutions in complex search spaces that would be impractical to solve using traditional optimization methods.
For instance, in autonomous vehicle control, AI can optimize vehicle behavior by adjusting steering, braking, and acceleration inputs based on real-time traffic and road conditions, ensuring both safety and efficiency.
4. Fault Detection and Diagnosis
Nonlinear systems are susceptible to a variety of faults that can degrade their performance. AI can play a significant role in detecting and diagnosing faults in real-time. By continuously monitoring system outputs and learning from past data, AI algorithms can identify deviations from normal system behavior and trigger corrective actions.
For example, AI-powered diagnostics are increasingly used in industrial automation to detect issues in machinery before they lead to system failure. In this way, AI enhances the reliability and longevity of nonlinear systems.
5. Predictive Control
AI techniques, particularly machine learning, can be integrated with predictive control methods to forecast future system behavior. Predictive control uses a model of the system to anticipate future states and adjust the control inputs accordingly. With AI, these predictions can be made more accurate by learning from data and continuously updating the system model.
This is particularly useful in complex systems such as smart grids, where future demand and supply of electricity must be predicted to optimize energy distribution. AI can learn from historical data and external factors to make more informed predictions and decisions.
Applications of AI in Nonlinear Control
-
Robotics In robotics, AI is used to control complex, nonlinear robotic systems, enabling them to perform precise tasks in dynamic environments. AI-based control systems help robots learn how to interact with their surroundings, adapt to changes, and make decisions that improve their performance.
-
Autonomous Vehicles Autonomous vehicles are a prime example of nonlinear systems, as they must continuously adapt to changing road conditions, traffic, and environmental factors. AI enables these vehicles to navigate through complex environments, make real-time decisions, and ensure safe operation.
-
Aerospace and Aviation In aerospace applications, nonlinear control systems are used to manage the dynamic behavior of aircraft and spacecraft. AI allows for more efficient flight path planning, real-time adjustments during flight, and improved safety through predictive control and fault detection.
-
Energy Systems In the energy sector, nonlinear control systems are used to optimize the generation and distribution of electricity, particularly in renewable energy systems like solar and wind power. AI helps manage fluctuations in energy production and demand, making energy systems more reliable and efficient.
-
Industrial Automation AI is transforming industrial automation by optimizing manufacturing processes, reducing energy consumption, and improving system efficiency. Nonlinear control systems in industrial settings can learn and adapt to new conditions, ensuring that production processes run smoothly with minimal downtime.
The Future of AI in Nonlinear Control
The integration of AI into nonlinear control is just beginning, and its potential is vast. As machine learning algorithms become more sophisticated and computational power increases, we can expect even more advanced and efficient control systems. Future advancements could lead to smarter autonomous systems, more accurate predictive control, and even more resilient infrastructure.
The use of AI in nonlinear control is expected to revolutionize industries ranging from manufacturing to transportation, healthcare, and beyond. By leveraging AI’s learning capabilities, engineers can create systems that are not only more efficient but also more adaptable, intelligent, and capable of handling complex, nonlinear dynamics.
Conclusion
AI-powered nonlinear control systems are transforming how we approach complex, dynamic systems in various industries. By harnessing the power of machine learning, neural networks, and optimization algorithms, AI is helping to overcome the challenges associated with controlling nonlinear systems. From robotics to energy systems, AI is unlocking new possibilities for performance, reliability, and adaptability.
To learn more about how AI is revolutionizing nonlinear control systems, watch the full video here:
As technology continues to evolve, the combination of AI and nonlinear control will pave the way for more intelligent, efficient, and autonomous systems, leading to advancements that could reshape industries and society as a whole.
Related Posts
AI for Nonlinear Control: A Revolutionary Approach
April 18, 2025 2025-04-11 10:37Popular Tags